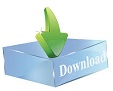

#Pixea for pics full
The device is fully reconfigurable and we demonstrate its use for the classification of handwritten digits from the MNIST dataset with an accuracy comparable to that achieved by readout of the full image, but with substantially lower delay and energy consumption. Each of these detectors can be addressed individually and their photoresponsivity values can be set by the application of a bias voltage. Here, we present a hardware implementation of this algorithm, based on a two-dimensional array of tunable metal–semiconductor-metal (MSM) photodetectors. SSPOC, on the other hand, has inspired applications in dynamics and control 24, 25, but has to the best of our knowledge not been employed in an imaging device yet. CS has likewise led to new types of image acquisition systems, such as single-pixel cameras 20, coded aperture imagers 21, and CMOS CS imaging arrays 22, 23. Many of those devices emulate certain neurobiological functions of the retina, either using complementary metal–oxide–semiconductor (CMOS) technology (silicon retina) 10, 11, 12, 13 or emerging device concepts 14, 15, 16, 17, 18, 19. Several new types of image sensors have been developed in recent years 9, targeting lower energy consumption and latency than their conventional frame-based counterparts. Subsequent samples can then be classified with performance comparable to that obtained by processing the full signal. In the sparse sensor placement optimization for classification (SSPOC) algorithm 7, 8, the data are not sampled randomly, but a few representative measurement locations are identified from training data. This can be achieved by using a task-specific basis, learned from data, instead of a generic one such as Fourier or Wavelet. Both sampling and compression are performed simultaneously to reduce the number of measurements at the expense of increased computational cost for signal reconstruction.īy combining CS with statistical learning, the number of required measurements can be further reduced, particularly if a given signal only needs to be assigned to one of a few categories, or classes, rather than being fully reconstructed. randomly) sampled at the encoder side, can be reconstructed at the decoder by finding the sparsest solution of an underdetermined linear system. In CS, a signal that is incoherently (e.g. Natural images, for example, are known to be sparse in the Fourier or Wavelet domains, which is exploited in several types of transform coding schemes, including the JPEG and MPEG standards 2.Ĭompressed sensing (CS) is a mathematical framework for the recovery of sparse signals from few measurements 3, 4, 5, 6. This can be understood from the fact that these signals may contain much redundant information or, more precisely, are sparse when represented in a proper domain or basis.

For many practical signals, however, reconstruction may still be possible from far fewer samples-or measurements-than required by the sampling theorem. The original signal can then be perfectly reconstructed from the samples. The Nyquist-Shannon sampling theorem 1 establishes a lower bound for the sampling rate required to capture any given signal of finite bandwidth without loss of information.
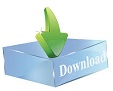